Is AI getting closer to human values?
It’s no secret that algorithms can produce systemically biased recommendations, such as in lending decisions. Some good news: A team at Anthropic tested the hypothesis that “language models trained with reinforcement learning from human feedback (RLHF) have the capability to ‘morally self-correct’ — to avoid producing harmful outputs — if instructed to do so.” The researchers found supporting evidence across three experiments, illuminating different facets of moral self-correction.
Highlights here. Paper available on Arxiv here (5 primary authors, 49 names total: It takes a village).
Some good news from models trained with RLHF. We’re getting a bit of transparency into how AI draws its conclusions, which is solid gold for advocates of Human+AI collaboration.
Open AI has explored the benefits of ChatGPT chain-of-thought prompts, which instruct a bot to explain its reasoning step by step, rather than simply providing an answer. Their recent paper offers excellent examples of the ‘process supervision’ method of rewarding models for providing step-by-step, chain-of-thought responses, vs the ‘outcome supervision’ method of rewarding final answers.
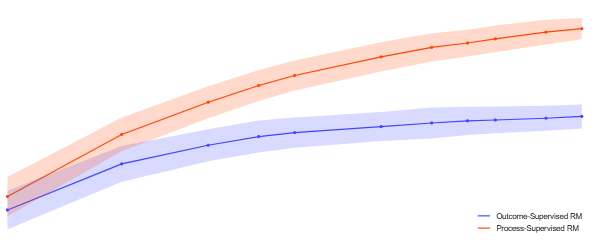
It gets better. Testing showed that getting human approval* of an LLM’s reasoning also signals ‘alignment’ (bot conformance to human values and judgments): “process supervision also has an important alignment benefit: it directly trains the model to produce a chain-of-thought that is endorsed by humans”. So far this has been tested using math problems/mathematical reasoning, much like “show your work” back in school. It will be a glorious day when chain-of-thought prompts reliably trigger step-by-step responses aligned with human values on public policy and other issues.
Museum of AI has lamented the disincentives (rarely rewards) for people to explain their decision methods. Often important ones are declared as an act of courage and leadership by an executive, unfortunately when the organization’s culture does not necessarily reinforce open dialogue and quality decision processes.
AI adoption may be a wake-up call for decision makers who hesitate to show any of their cards. Sure, strategic decisions require more than a play-by-play of the evidence and thought process ~ spin can encourage necessary buy-in. But as AI gains strength at recommending, and slowly learns to explain ~ humans need to do the same.
*But wait. Which humans?
Human values aligned with ChatGPT responses? Sounds awesome. But how are conflicting (human) values aggregated or otherwise resolved? This is the age-old problem with weighing preferences, whether they’re stated by one or 100,000 humans. See previous posts on how human-in-the-loop AI and multicriteria decision analysis are like HouseHunters. Also a short but sweet Twitter conversation referencing Kenneth Arrow (he of the 1963 theorem) and how to handle social preferences. @xuanalogue is definitely worth following.